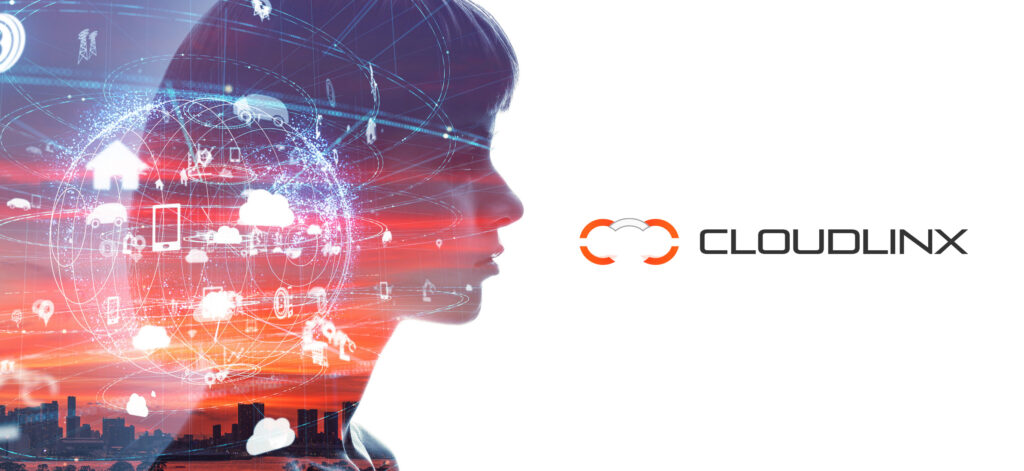
Deploying generative AI for unsuitable business use cases often results in high failure rates and reduces its overall value within organizations. To prevent this, CX leaders can refer to this guidance to assess whether GenAI is the right solution for their needs or if other AI methods might be more appropriate.
Overview
Key Findings:
CX leaders often struggle to determine when generative AI (GenAI) is the right solution for their use cases. The hype surrounding GenAI can lead to its application in situations where it’s not the best fit, increasing the risk of unnecessary complexity and potential project failure. An overemphasis on GenAI may also cause CX leaders to overlook other AI techniques that might be more effective for certain tasks, reducing overall business value.
AI techniques aren’t mutually exclusive; in fact, they can often be integrated to create a more powerful system. Organizations that learn to combine the right AI methods are better positioned to develop solutions with enhanced accuracy, transparency, and performance, all while reducing costs and minimizing data requirements. This integrated approach helps unlock the full potential of AI to address a wide range of business challenges.
Recommendations:
Categorize and Evaluate Use Cases
Systematically categorize each use case to assess its suitability for generative AI (GenAI).
Use cases related to prediction and forecasting, planning and optimization, decision intelligence, and autonomous systems are not currently ideal for GenAI models in isolation.
Consider Alternative AI Techniques
Utilize alternative AI methods when GenAI is not the best fit. Prominent alternatives include nongenerative machine learning (ML), optimization, simulation, rule-based systems, and graphs. These techniques are often more reliable and better-understood than GenAI for many use cases.
Combine GenAI with Other AI Techniques
Integrate GenAI with other AI methods to build stronger, more robust systems. Combining GenAI with other techniques can address its limitations, such as its propensity for inaccuracies and hallucinations. Alternatively, use GenAI models to enhance other AI systems, such as leveraging them as a natural language interface for AI and software tools.
Introduction:
Generative AI (GenAI) adoption has surged in recent months, with organizations across industries rapidly integrating it into various business use cases, from content creation to customer service. While this rapid rise is promising, it also brings the risk of overshadowing the broader AI landscape. For many businesses, particularly those new to AI, GenAI may be their first exposure to the technology, leading them to equate GenAI with AI in general. This narrow view could prevent organizations from exploring other AI methods that might be better suited to specific challenges.
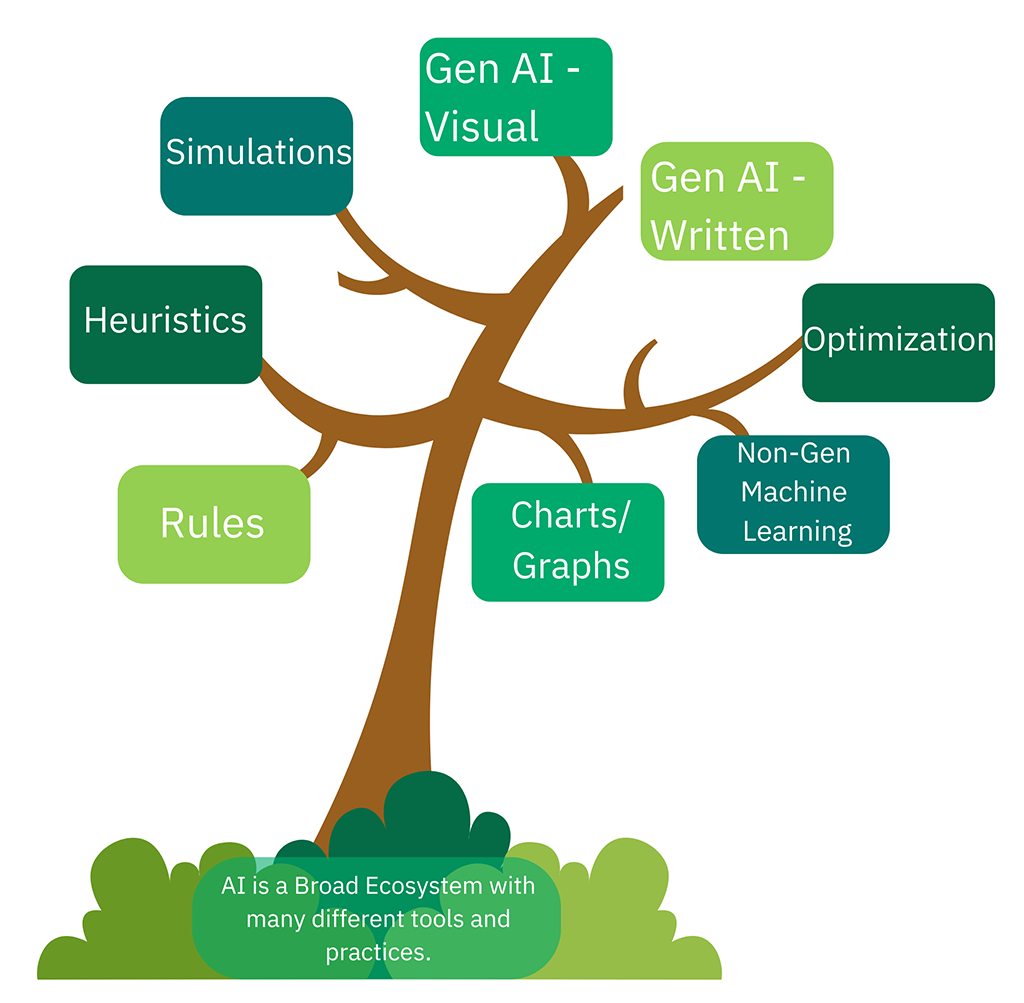
While GenAI is undeniably a powerful tool, it is just one component of a much broader AI ecosystem. Techniques like machine learning, optimization, and rule-based systems can often provide more reliable, transparent, and effective solutions for specific use cases, such as predictive analytics and decision-making. By focusing exclusively on GenAI, organizations risk missing out on opportunities to leverage these other AI methods, which could drive greater business value. A more balanced approach—one that combines GenAI with other AI techniques—will enable businesses to create more robust, efficient, and adaptable AI systems.
This issue is particularly important because different use cases often require different AI techniques. GenAI is not a one-size-fits-all solution and may not be the best fit for many AI challenges. Many business problems require a mix of various AI methods, which organizations may overlook if they focus too narrowly on GenAI. The growing hype around GenAI can also lead to unrealistic expectations, as many of these technologies are still in the “Peak of Inflated Expectations” phase. As a result, CX leaders risk deploying GenAI in situations where it doesn’t provide the best solution, which can lead to overestimated potential and underestimated complexity. To avoid such pitfalls, CX leaders should carefully assess when GenAI is unsuitable for a given use case, identify when other AI techniques might be more appropriate, and determine how to combine them effectively for optimal results.
Analysis
Evaluate Whether GenAI Is Right for Your Use Case
Deciding whether to use GenAI models should be done on a case-by-case basis. The first step is to ensure that the use case itself is valuable and feasible, regardless of the AI technique being considered. Some use cases may not be suitable for AI at all and shouldn’t be pursued further. A useful approach is to prioritize and evaluate potential use cases using a structured framework or tool to help identify which ones hold the most promise.
Once use cases are prioritized, map them to relevant categories to assess the potential effectiveness of GenAI models. By organizing use cases into distinct families, you can better understand where GenAI is most applicable. This method provides a clearer picture of where GenAI might offer the most value and helps simplify the often-complex landscape of AI use cases.
There are several use-case families where GenAI models are commonly misapplied within organizations (marked as “Low” in Table 1):
Prediction and Forecasting:
It is currently a mistake to rely on GenAI models, such as large language models (LLMs), for tasks like demand prediction, sales forecasting, time-of-arrival estimation, weather forecasting, or supply chain forecasting. LLMs are not designed for numerical predictive modeling or statistical tasks like regression, which is required to predict continuous variables based on input features. While LLMs may help generate features for these tasks, the forecasting itself should be handled by alternative AI techniques more suited for numerical predictions.
Planning:
GenAI models fall short when it comes to planning and optimization tasks, which are crucial for use cases such as inventory optimization, workforce scheduling, route planning, financial portfolio management, pricing optimization, and resource allocation. These tasks demand precise calculations and optimization, areas where current GenAI models are not strong. Attempts have been made to integrate LLMs into broader planning systems, but they do not yet match the capabilities of traditional AI methods in optimization and planning.
Decision Intelligence:
Decision intelligence uses AI to support complex decision-making processes, such as choosing the best course of action to achieve a desired outcome. GenAI models are not built for decision-making tasks— their outputs are unreliable, lack explainability, and cannot model decisions effectively. It is risky to use GenAI for critical decisions in areas like hiring, financial planning, supply chain management, and strategic decision-making. Instead, LLMs may be used as a conversational interface for systems that handle actual data analysis.
Autonomous Systems:
GenAI models currently have a significant “autonomy gap,” meaning they are not robust enough to operate independently and require human supervision due to issues like inaccuracies and hallucinations. While there are ongoing efforts to develop LLM-based agents, these systems are still limited to narrow domains and often rely on a “human in the loop.” This makes GenAI unsuitable for high-stakes use cases like industrial robotics, autonomous vehicles, and algorithmic trading.
Additionally, GenAI might not be suitable for your use case if the risks associated with its use outweigh the potential benefits.
GenAI poses specific risks, including unreliable outputs, data privacy concerns, intellectual property issues, liability, cybersecurity vulnerabilities, and regulatory compliance challenges. These factors need to be carefully evaluated for each use case (see Tool: Generative AI Policy Kit).
Over time, as GenAI techniques improve and are combined with other AI methods, their effectiveness for these use cases may evolve. However, for now, CX leaders should consider exploring alternative AI approaches, which are discussed in the following section.
Consider Alternative AI Techniques to GenAI
The growing focus on GenAI has diverted attention from other established and effective AI techniques. In many cases, alternative AI methods—or a combination of them—may be better suited to support specific use cases.
By evaluating your use case against different AI techniques, you can identify the most appropriate approach for your needs. While GenAI has its strengths, other techniques may be more effective as the primary solution for certain challenges. Exploring a combination of methods can further enhance the capabilities and outcomes of your AI systems.
While not an exhaustive list, the following represents some of the most widely used AI techniques. Other emerging methods—such as causal AI, neuro-symbolic AI, and first-principles AI—are also gaining traction and are worth monitoring as they evolve.
The saying “If all you have is a GenAI hammer, everything looks like a GenAI nail” highlights the danger of over-relying on GenAI for all AI needs. Here are some key alternative techniques to consider:
Nongenerative ML (Predictive ML):
This approach involves using machine learning models trained on historical data to make predictions, such as categorizing images or predicting continuous variables (e.g., customer lifetime value). Nongenerative ML has been the dominant AI approach for the last decade, excelling in forecasting, customer segmentation, anomaly detection, recommendation systems, and predictive maintenance. For many use cases, these models are more suitable than GenAI.
Optimization:
Commonly used in operations research, optimization techniques focus on maximizing benefits while balancing trade-offs between business objectives. These techniques are ideal for planning use cases like inventory management, workforce scheduling, route planning, pricing strategies, and financial portfolio management. Optimization can also enhance decision intelligence and autonomous systems, making it more effective for these tasks than GenAI.
Simulation:
Simulations replicate real-world scenarios to support decision-making by testing different outcomes without actual implementation. They are used in applications like financial modeling, strategic planning, supply chain simulations, and autonomous vehicle training. Simulations offer a more controlled and explainable approach to prediction, decision intelligence, and content generation than GenAI.
Rules/Heuristics:
Rule-based systems capture expert knowledge in the form of rules to aid decision-making, such as in fraud detection, medical diagnosis, and risk assessment. These systems are highly interpretable, making them a preferred choice for sensitive use cases where explainability is essential, unlike GenAI models, which can lack transparency.
Graphs:
Knowledge graphs represent entities (e.g., customers, products) and their relationships in a network of nodes and links. They are especially useful for tasks requiring clear data retrieval and explainability, such as recommendation systems, fraud detection, search engines, and data management. Graphs can also be combined with GenAI to add verified, trusted data to their outputs.
These alternative techniques can be more efficient, reliable, and easier to understand than GenAI for many use cases. When deciding on the right approach, it’s important to consider factors like explainability, performance, and reliability.
Since GenAI models tend to be less predictable and harder to interpret, starting with a simpler, more transparent AI technique can reduce risk, lower costs, and provide clearer insights.
Combine GenAI Models with Other AI Techniques
AI techniques are not mutually exclusive; in fact, they can often be integrated to create more powerful and effective systems. The most advanced AI solutions typically combine a variety of techniques to enhance accuracy, transparency, and performance while also reducing costs and minimizing data requirements. By leveraging multiple AI methods together, organizations can build more robust systems that address a broader range of challenges.
For many use cases, combining AI techniques may provide the best solution. Pairing GenAI with other methods can help mitigate some of its limitations, such as its tendency for inaccuracies and hallucinations. For example, integrating knowledge graphs with large language models (LLMs) can significantly improve the reliability of outputs by reducing the occurrence of hallucinations. This approach allows businesses to take advantage of the strengths of both techniques, creating more accurate and trustworthy AI systems.
Additionally, GenAI can be used to enhance traditional AI techniques. LLMs, for example, can help build and interact with knowledge graphs, facilitating easier access to and management of data. GenAI can also serve as a natural language interface to other AI systems, improving their accessibility and user-friendliness. Furthermore, GenAI can generate features that can be fed into other AI models, such as those based on nongenerative machine learning. The possibilities for combining these AI techniques are vast, with many practical applications ranging from knowledge graphs to analytics and business intelligence systems.
Conclusion
As organizations continue to explore the potential of Generative AI (GenAI), it’s important to recognize that it is not a one-size-fits-all solution. While GenAI offers exciting possibilities, it is not always the best fit for every use case. In some cases, alternative AI techniques—such as nongenerative machine learning, optimization, simulation, rule-based systems, and knowledge graphs—may offer more reliable, efficient, and interpretable solutions. Understanding when and where to apply GenAI, and when to turn to other techniques, is critical for maximizing the value AI can bring to the organization.
The most effective AI systems often combine different techniques to create more robust solutions.
GenAI can be integrated with established methods to overcome its limitations, like inaccuracies and lack of robustness, while also enhancing the capabilities of traditional AI approaches. For instance, combining GenAI with knowledge graphs or using it as a natural language interface for other AI systems can provide more transparency and improve overall performance.
Ultimately, organizations should take a strategic approach to AI adoption, evaluating each use case carefully to determine the most suitable technique or combination of techniques. By doing so, they can mitigate risks, optimize outcomes, and avoid the pitfalls of over-relying on a single AI method.